The financial industry is witnessing a significant transformation as artificial intelligence, particularly through the adoption of Large Language Models (LLMs), becomes a pivotal element in banking operations. Banks are leveraging the advanced capabilities of LLMs to not only enhance customer service but also to streamline complex processes, analyze massive datasets, and ultimately make more informed business decisions. The interaction between AI and finance signifies a shift towards more efficient, personalized, and innovative financial services, illustrating why institutions like JPMorgan are investing in AI technologies.
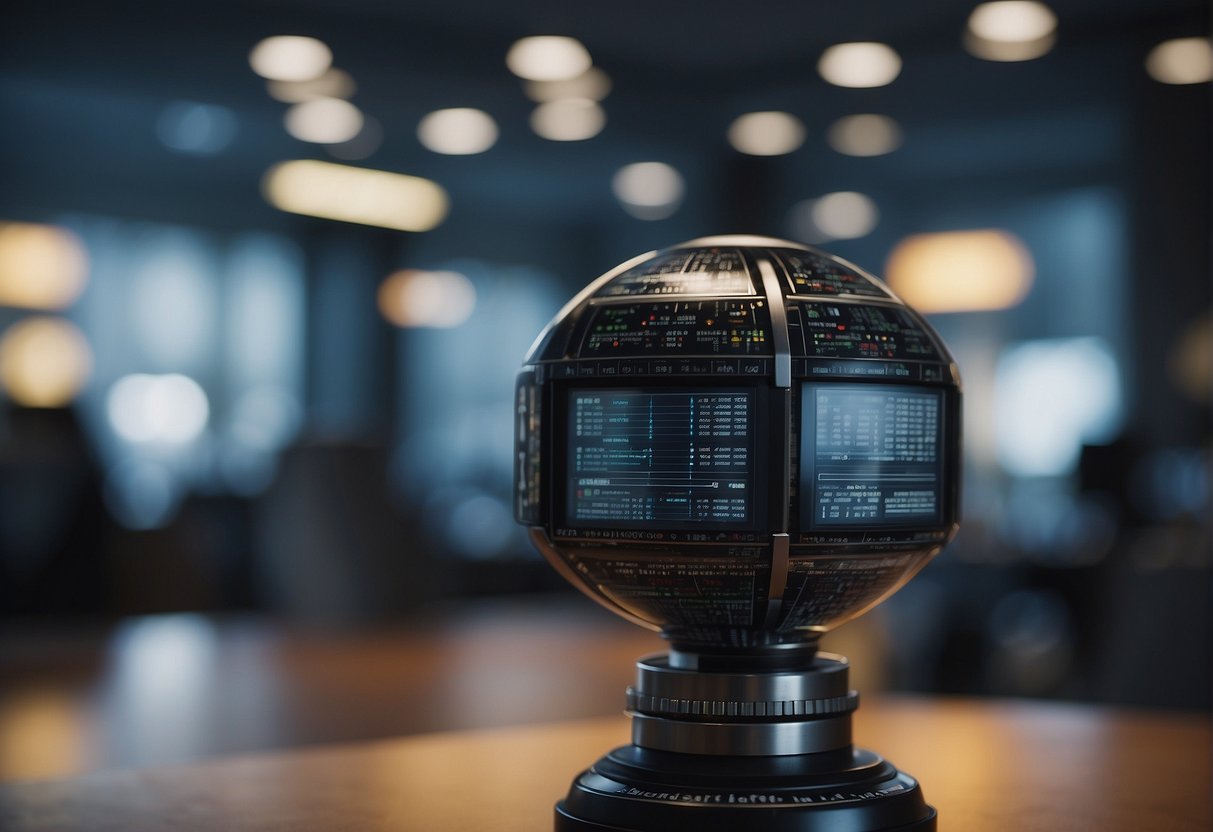
The implementation of LLMs in finance is reshaping the customer experience, with AI-driven platforms providing natural language interactions that mirror human conversation. This development paves the way for customers to receive instant, intelligent responses to their inquiries, making banking more accessible and user-friendly. Moreover, the back-end advantages are substantial; LLMs can automate and optimize workflows, detect fraudulent activities, and deliver predictive analytics, thus allowing banks to operate with greater accuracy and reduced risk.
Financial giants are recognizing the transformative impact of AI as it extends into various sectors of finance, from wealth management to regulatory compliance. With AI proving to be a versatile tool, banks are exploring the full breadth of its applications to maintain a competitive edge. This embrace of technology highlights an industry at the cusp of a tech-led evolution, with LLMs at the forefront as a key driver of change.
Evolution of AI in Finance
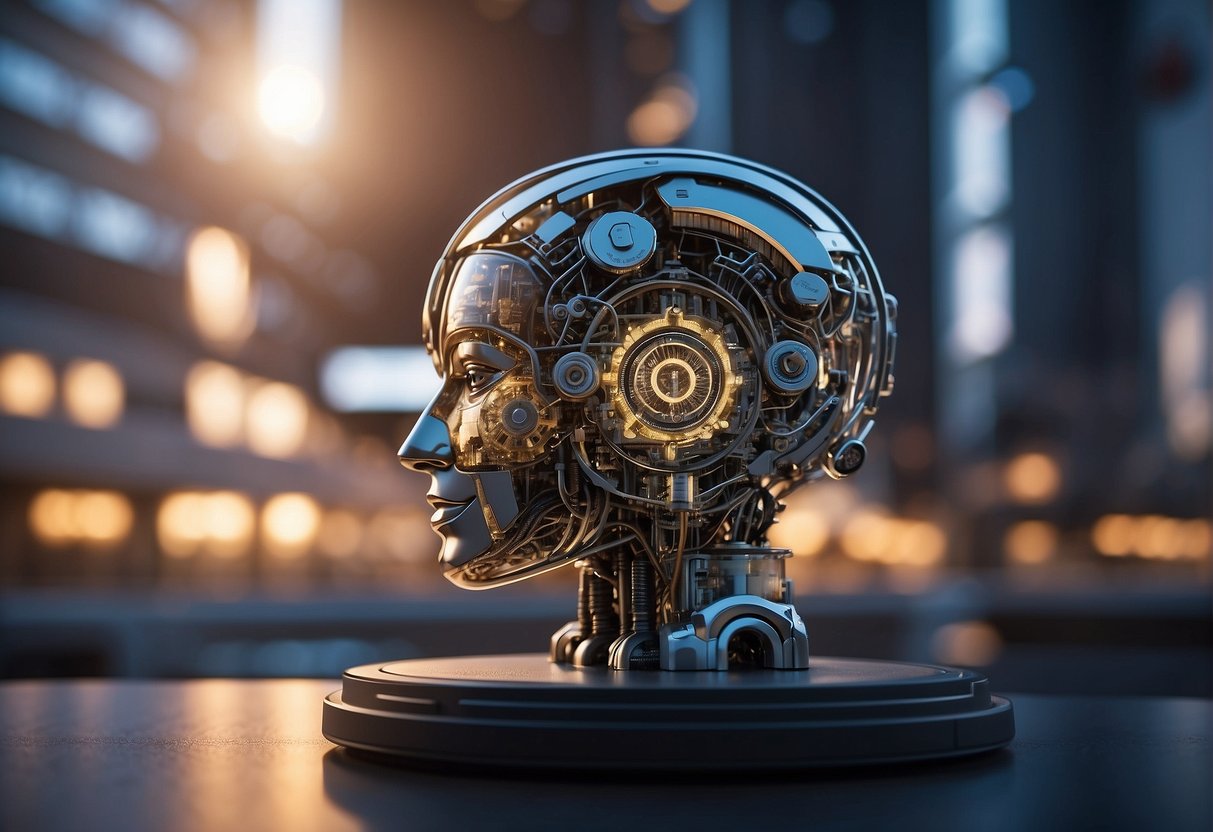
The financial industry has been witnessing a significant transformation with the integration of AI, particularly as banks embrace language model technologies and AI-driven processes.
Historical Context
Initially, Artificial Intelligence in finance focused on automating simple tasks, such as calculations and data analysis. Financial institutions quickly recognized the potential of AI to perform more complex operations, such as fraud detection and risk management. In the early stages, AI systems were rule-based and lacked the ability to learn from data. However, as machine learning techniques matured, these systems began to adapt and evolve, leveraging historical data to improve their accuracy and functionality.
Current Developments
Today, financial entities are harnessing sophisticated AI technologies, including language models, to transform customer service and streamline operations. Through AI, services have become more personalized, with technologies such as AI in banking playing a pivotal role in analyzing customer data to offer targeted financial products. Compliance and security have also experienced advancements, with AI enabling real-time transaction monitoring and anomaly detection to prevent fraud. Moreover, the adoption of AI by banks is noted for creating strategic partnerships, as outlined in discussions surrounding AI’s influence on finance and banking.
Adoption of Language Models by Banks
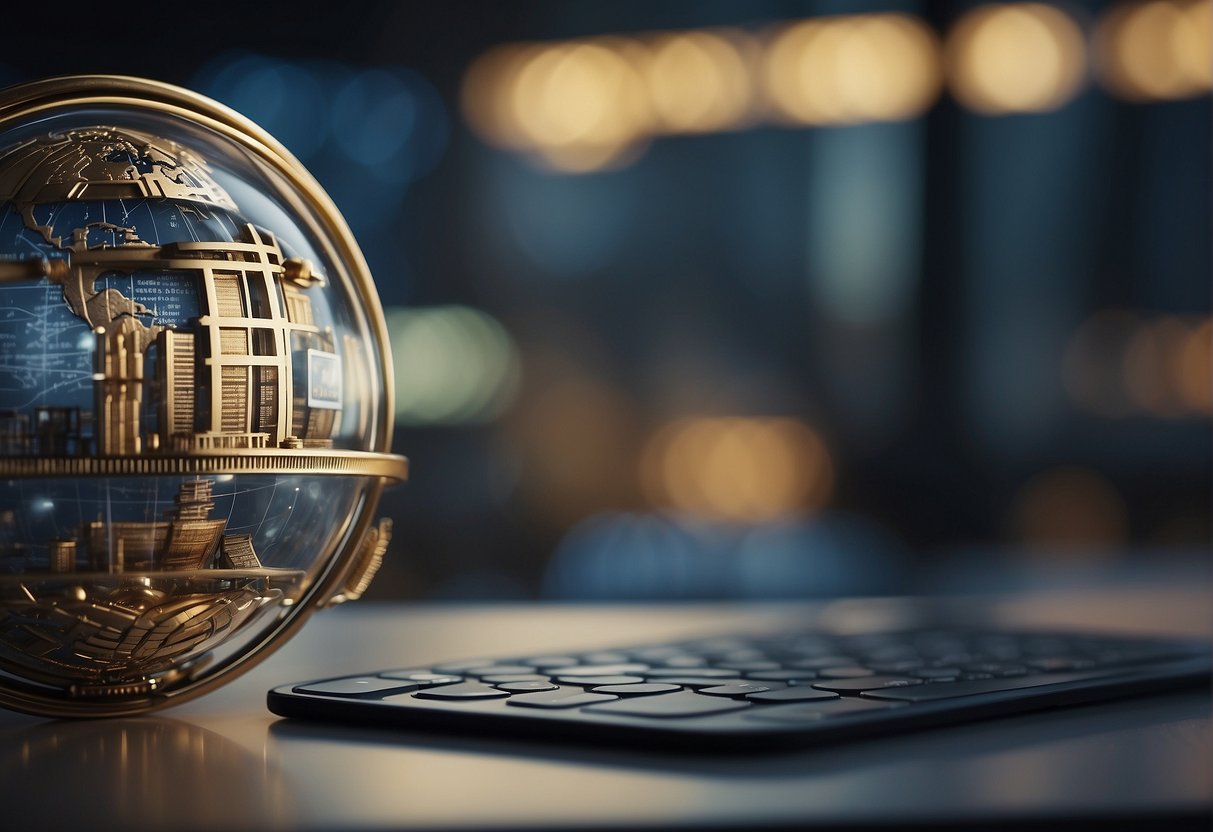
Financial institutions are rapidly integrating large language models (LLMs) into their operations, recognizing their potential to transform customer experience and streamline internal processes.
Areas of Implementation
- Customer Service: Banks leverage LLMs for enhancing customer interactions through chatbots and virtual assistants, providing timely and efficient responses. An example is Morgan Stanley Wealth Management, which utilizes data to improve service quality.
- Risk Management: These models are instrumental in monitoring and analyzing risks by processing vast datasets, which aids in informed decision-making.
- Compliance: The automation of compliance checks and regulatory reporting is another key area where LLMs are being deployed to ensure adherence to legal standards.
- Fraud Detection: With the ability to analyze patterns within large datasets, LLMs significantly enhance the detection and prevention of fraudulent activities.
Banks are embracing these innovations, betting on the technology to deliver a competitive edge.
Impact on Financial Services
- Operational Efficiency: By automating routine tasks, LLMs are significantly reducing the operational costs and time for banks.
- Customer Experience: Personalized banking experiences are becoming a reality as LLMs offer tailored advice and support, leading to higher customer satisfaction.
- Innovation: The introduction of LLMs is fostering innovation within financial services, encouraging the development of new products and services.
The application of LLMs is demonstrating measurable improvements in accuracy and customer engagement, evident in the Bank of England/FCA 2022 survey on ML adoption, highlighting the substantial percentage of firms utilizing or developing ML applications.
Through these targeted implementations, banks are setting new benchmarks in operational excellence and customer service.
Technological Advancements in AI
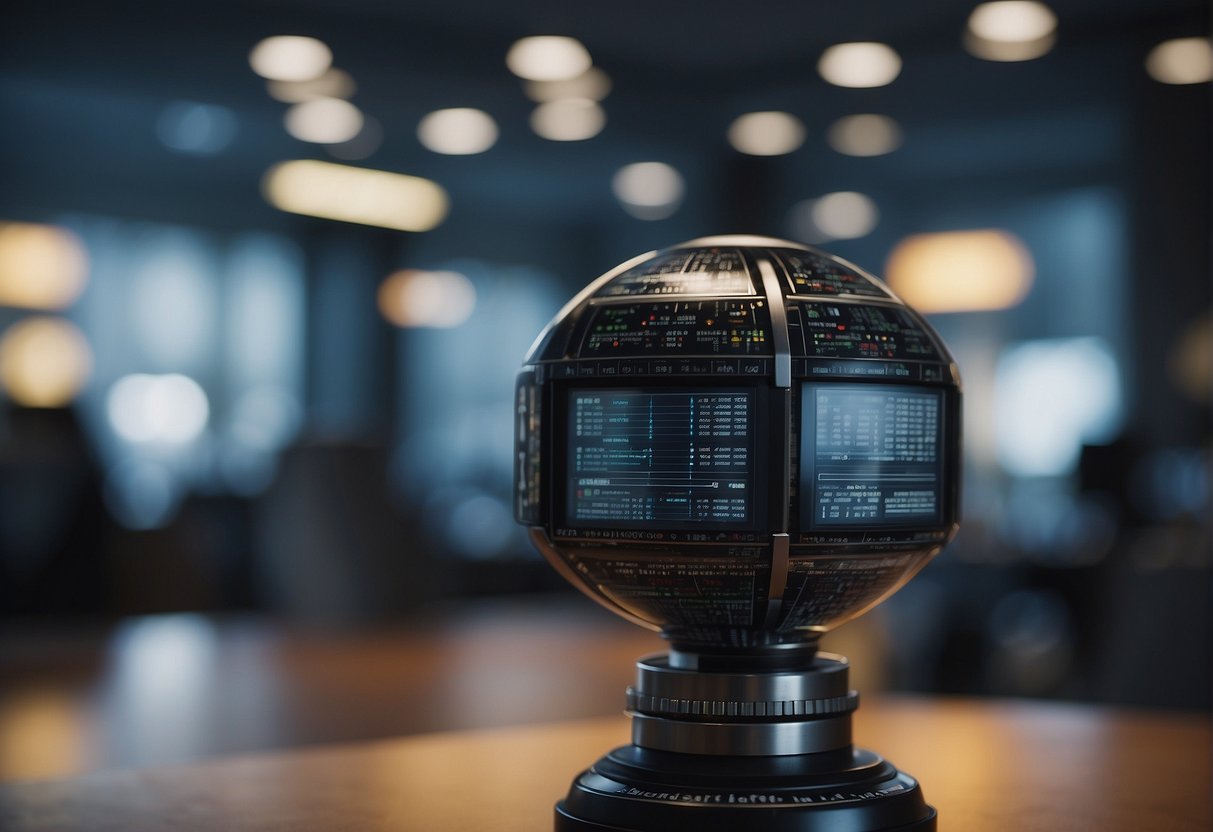
The finance industry witnesses a significant shift as banks integrate Large Language Models (LLMs) to revolutionize services and operations. These advancements enhance both customer experience and backend efficiency.
Language Model Improvements
Recent enhancements in Language Models signify a leap in natural language understanding and generation. For instance, newer LLMs have reduced errors in comprehension tasks, resulting in more accurate financial advice and risk assessment. Investments in these technologies are seen as pivotal steps for banks to stay competitive in a rapidly evolving digital landscape.
AI and Computational Finance
In computational finance, AI is increasingly relied upon to analyze large datasets and identify market trends. Banks leverage sophisticated algorithms for real-time processing of financial transactions, enhancing their decision-making processes and fraud detection capabilities. The integration of AI-driven systems into trading strategies also offers optimized asset allocation and predictive analytics.
Regulatory Considerations for AI in Finance
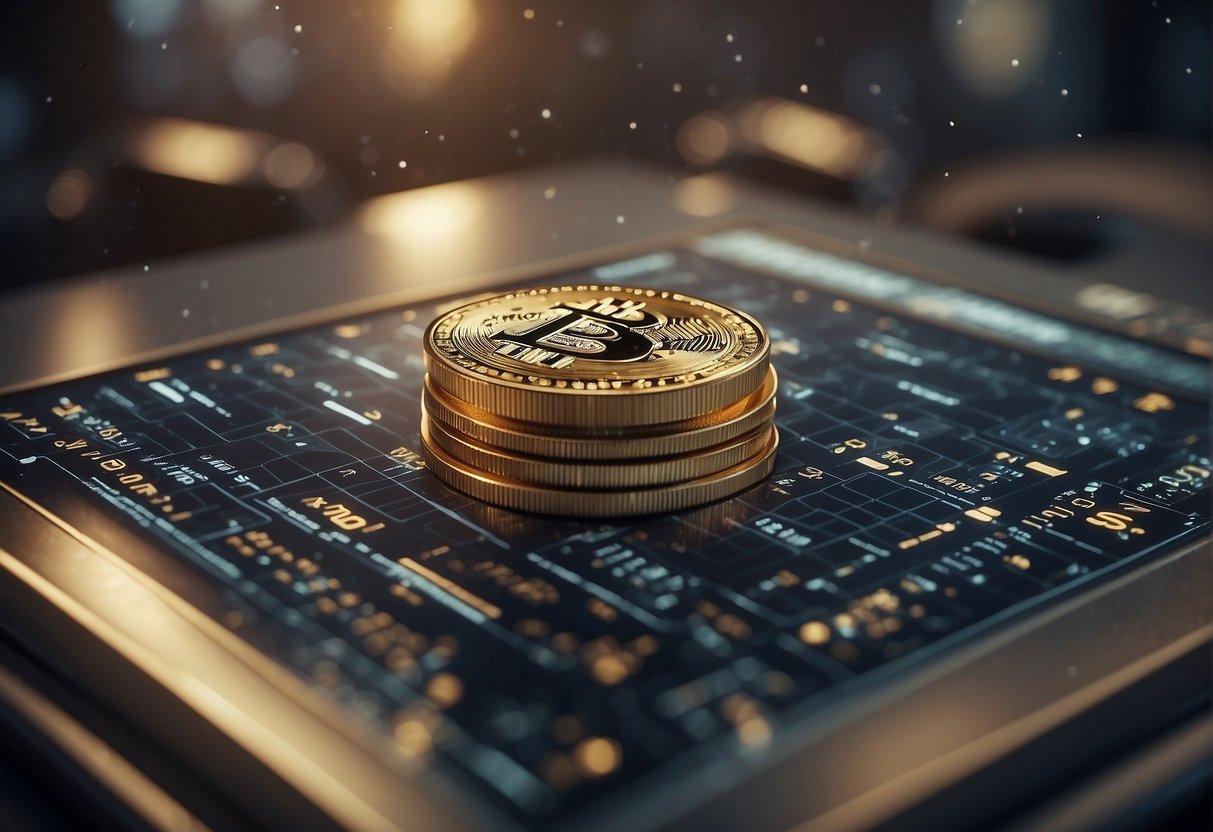
The expansion of AI in financial services raises complex compliance issues and has drawn regulatory attention. Institutions must navigate these challenges to benefit fully from AI’s potential while adhering to evolving regulations.
Compliance Challenges
Financial institutions integrating AI face significant compliance hurdles. They must ensure any AI solutions—including large language models (LLMs)—comply with a myriad of regulations concerning consumer protection, privacy, and fair lending. One primary concern is the explainability of AI decisions, which can be opaque in complex models. Ensuring that decision-making is transparent and nondiscriminatory is essential, particularly when AI is used for credit scoring or risk assessments.
Institutions also grapple with data governance issues, as AI systems require extensive data to function effectively. They must enforce strict data security measures and manage data quality to prevent biases in AI outputs.
Regulatory Responses
Regulators have started to address the adoption of AI in the financial sector with guidelines and consultation papers that clarify existing rules. Recent regulatory activity emphasizes the importance of institutions maintaining rigorous oversight of AI technologies. In the United States, for instance, responses to the risks associated with AI have included clarifications around Model Risk Management (e.g., SR 11-7).
Internationally, bodies are calling for heightened responsibility regarding AI deployment. In particular, financial institutions are reminded that they are liable for ensuring AI compliancy with the law, as noted by officials from various agencies including the Federal Reserve and the Office of the Comptroller of the Currency, emphasizing the institutions’ ultimate responsibility even when AI applications are contracted through third parties.
Financial services are wise to proactively adhere to these regulatory standards to avoid the legal and reputational risks associated with non-compliance.
Future of AI in Finance
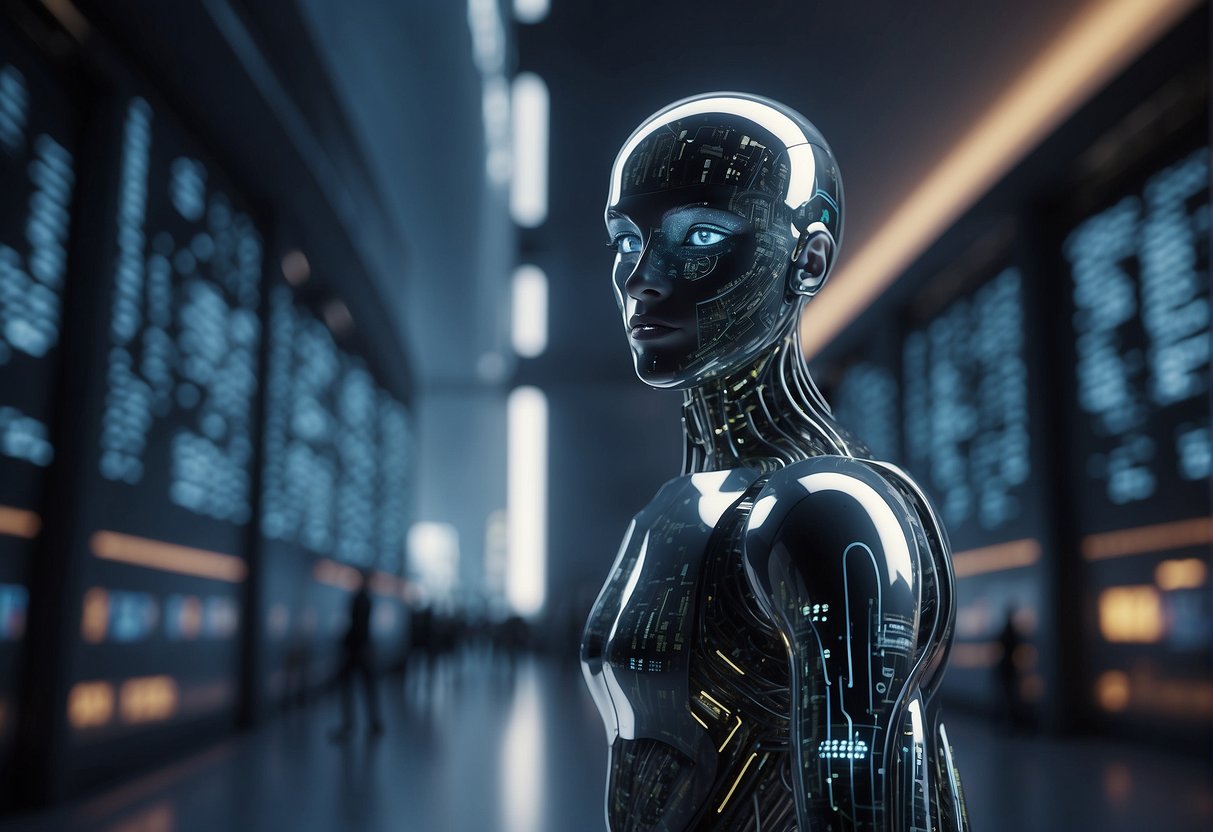
Artificial intelligence (AI) is reshaping the financial sector by enabling enhanced predictive analytics and offering personalized banking services that were once the realm of science fiction. Banks are harnessing the power of AI to transform data into actionable insights and fine-tune their interactions with clients.
Predictive Analytics
Predictive analytics in finance takes past data to forecast future events. By leveraging machine learning algorithms, financial institutions can predict market trends, identify investment opportunities, and manage risks with greater precision. For example, AI systems can analyze years of market data to anticipate stock performance patterns, helping to guide investment strategies with enhanced foresight. McKinsey estimates that deploying AI technologies could potentially add up to $1 trillion of additional value each year to global banking. Despite these advancements, scaling AI technologies remains a challenge due to factors such as the lack of clear AI strategy and inflexible legacy systems.
Personalization of Banking Services
Personalized banking services have evolved with AI’s capability to aggregate and analyze customer data. Financial institutions are now providing customers with individualized recommendations, tailored investment advice, and bespoke financial products. A concrete example is the personalized budgeting advice tools that assess an individual’s spending patterns and offer tailored suggestions for saving money. AI-fueled systems aim to improve client satisfaction by delivering a service that feels bespoke and comprehensive, reflecting the customer’s unique financial landscape.
The finance industry’s implementation of AI not only promises to revolutionize the way services are delivered but also to redefine the customer-bank relationship through a more personalized, tech-driven approach.
Challenges in Integrating LLMs
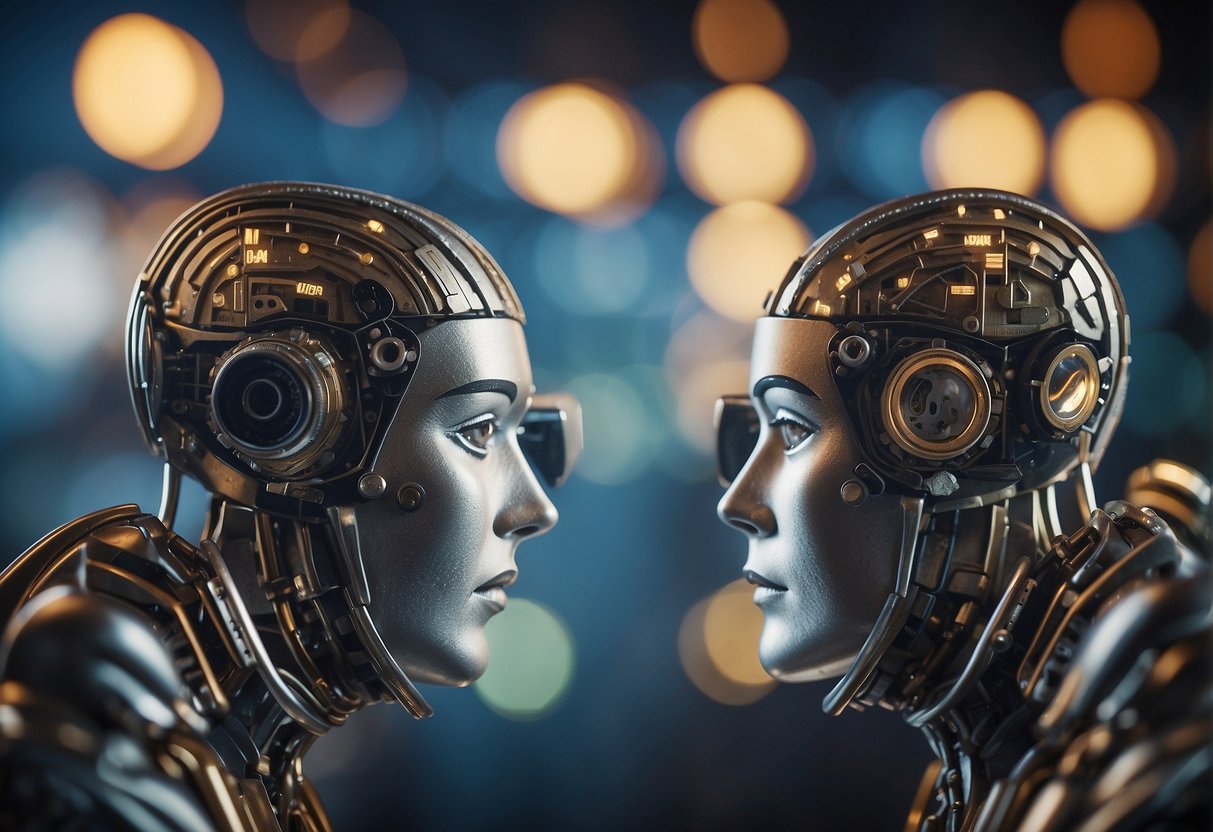
Integrating Large Language Models (LLMs) into the financial sector presents specific hurdles that institutions must navigate cautiously.
Data Privacy Concerns
The use of LLMs in the banking industry necessitates handling vast amounts of sensitive data, raising significant data privacy concerns. Financial institutions are bound by strict regulations like the General Data Protection Regulation (GDPR) and the California Consumer Privacy Act (CCPA), which mandate rigorous data protection measures. Ensuring that LLMs comply with these regulations without compromising functionality is a delicate balance to maintain.
Addressing Bias and Fairness
Another pressing challenge is the presence of inherent biases within LLMs, which can lead to unfair treatment of customers. These biases often stem from the historical data on which the LLMs are trained. Banks must work to identify and mitigate these biases to ensure that their AI-based offerings are fair and non-discriminatory, which is not only a regulatory requirement but also a cornerstone of ethical banking practice.
Frequently Asked Questions
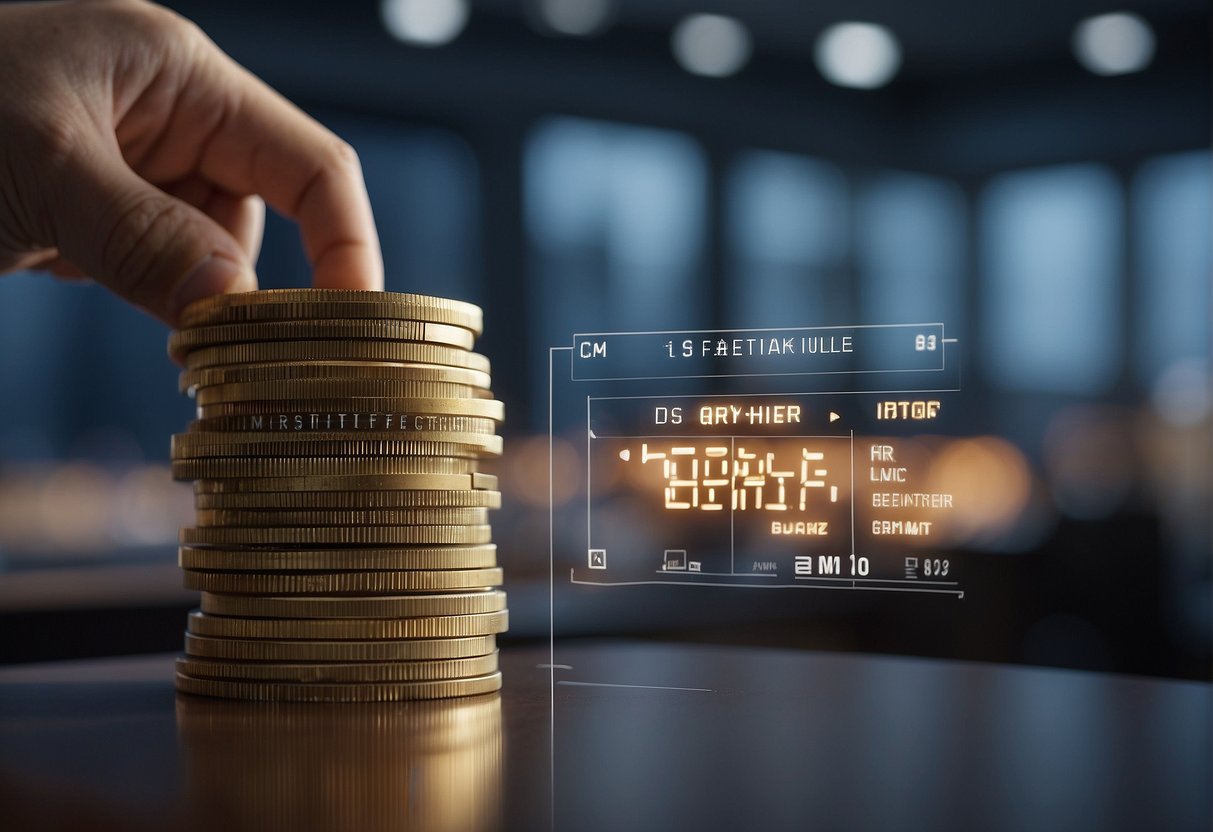
The integration of AI into the banking and financial sectors marks a significant shift towards more efficient and personalized services. This section addresses common inquiries regarding the impact and implementation of artificial intelligence in these industries.
How will AI impact the future landscape of the banking and financial sectors?
AI is poised to revolutionize banking by introducing advanced data processing and customer service personalization. It’s expected to unlock new operational efficiencies and foster innovative financial products and services.
Could AI pose a risk to the stability or employment within traditional banking institutions?
While AI could lead to increased efficiency, there is a valid concern that it may disrupt current banking jobs. However, many experts suggest AI will create new roles centered on AI management and oversight, thus potentially balancing out employment shifts.
What are the potential drawbacks of integrating AI into banking operations?
Some potential drawbacks include concerns over data privacy, increased vulnerability to sophisticated cyber attacks, and the ethical implications of using AI to make decisions that have significant financial impacts on customers.
In what ways is artificial intelligence currently utilized within the financial industry?
Artificial intelligence is currently deployed in fraud detection, risk management, customer service via chatbots, and personalized financial advisory services, substantially enhancing the industry’s efficiency and client engagement.
What strategies are financial institutions adopting to incorporate large language models into their services?
Financial institutions are implementing large language models to automate customer support, drive conversational banking platforms, and generate insights from large volumes of data to support decision-making processes.
How might customer experiences in banking be transformed by the application of AI technologies?
AI technologies are expected to make banking more accessible through 24/7 automated customer service, provide tailored financial advice, and streamline processes such as loan approvals, making them faster and more user-friendly.
- AI in Retail: Transforming Customer Experience and Personalization Effortlessly
- Responsible AI Development: Ethical Adoption and Transparency
- AI and Creativity: The Fusion of Art, Music, and Machine Learning
- AI-Powered Chatbots in Customer Service
- Visa Bets on Web3 Loyalty
- AI is Eating Finance: How Banks are Embracing Large Language Models